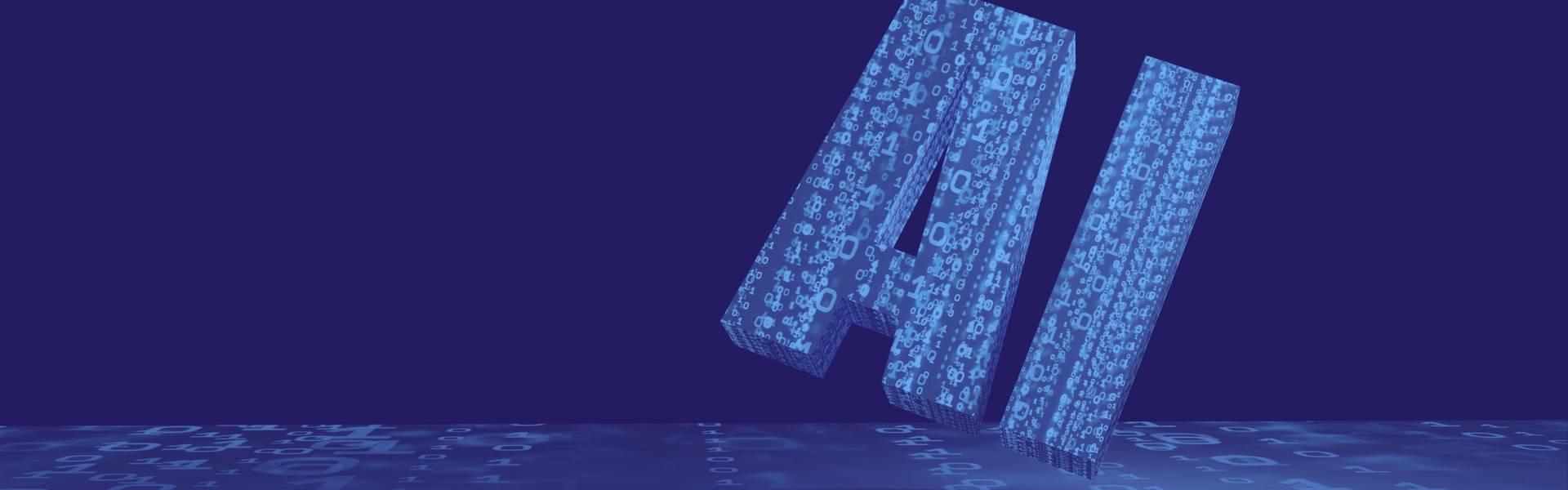
Generative AI: Top Challenges Hindering Its Adoption
Generative AI (Gen AI) is poised to revolutionize industries globally. From accelerating drug discovery in healthcare to crafting hyper-personalized marketing campaigns, its potential is undeniable. However, C-suite leaders must navigate key challenges to unlock Gen AI's true ROI and ensure responsible integration.
Based on our experience, this article dives into the five main hurdles hindering widespread Gen AI adoption and offers actionable strategies for overcoming them.
Challenges to Gen AI Adoption
Challenge 1: Data Dilemmas
Training robust Gen AI models requires vast and diverse datasets. However, securing high-quality, domain-specific data can be a bottleneck. Imagine a retail giant struggling to find a comprehensive dataset encompassing customer demographics, buying habits, and social media trends.
Solution: Building a Data-Centric Culture
- Partner with data science vendor agnostic experts to refine data collection strategies.
- Leverage synthetic data generation techniques to augment existing datasets.
- Manage data through a data fabric for a unified and consistent view of data across distributed environments.
Challenge 2: How do you explain it?
The lack of explainability in Gen AI models is a major concern. Businesses, particularly in sectors like finance or healthcare, require transparency in decision-making. How can you trust a model that generates financial forecasts without understanding the underlying rationale?
Solution: Demystifying the Machine
- Implement interpretable machine learning models that provide insights into decision-making processes.
- Leverage techniques like feature importance analysis and visualizations to understand model behavior.
- Prioritize user-friendly explainability interfaces for human oversight and audit.
Challenge 3: The Power Struggle
Training and deploying Gen AI models, especially deep learning architectures, requires significant computational resources. Imagine a startup in the manufacturing sector struggling to afford the computing power needed for a complex product design system.
Solution: Embracing the Cloud and Quantum
- Explore cloud-based solutions offering on-demand, scalable computing power.
- Stay updated on advancements in quantum computing, which holds immense promise for accelerating Gen AI model training and processing.
Challenge 4: The Legal Labyrinth
The legal and regulatory landscape surrounding Gen AI is still evolving. Issues like intellectual property rights, data privacy, and liability pose challenges. For instance, the entertainment industry grapples with copyright ownership when Gen AI generates music compositions.
Solution: Advocating for Clear Frameworks
- Collaborate with industry leaders and policymakers to establish clear legal and regulatory frameworks for Gen AI.
- Stay informed about evolving regulations and ensure compliance across all Gen AI initiatives through Industry National Associations and other official entities.
Challenge 5: The Ethical Imperative
The potential misuse of Gen AI for generating deepfakes, spreading misinformation, or creating biased content is a significant concern. Imagine the reputational damage a biased AI model could inflict on a financial institution's lending practices.
Solution: Prioritizing Ethical AI
- Implement robust human oversight and control mechanisms to ensure responsible Gen AI usage.
- Prioritize fairness and inclusivity throughout the development and deployment process.
- Foster a culture of transparency and accountability within your organization.
By addressing these challenges, businesses can pave the way for the successful adoption of Gen AI. Partnering with experienced AI solution providers can offer invaluable expertise in navigating these complexities. Together, you can unlock the true potential of Gen AI, driving innovation, boosting productivity, and achieving a significant competitive advantage.
Contact us today to leverage our team's skills and 18+ years of cross-sector experience in developing AI models. We deliver tailored solutions that align with our client's unique requirements and drive value across their operations.